About
AI-driven segmentation models hold great promise for improving clinical outcomes in radiology but MRI images vary greatly across different hospitals, scanners, and clinical settings. This variability, known as "acquisition shifts," can reduce AI reliability, creating risks in clinical practice. How can healthcare professionals ensure AI models remain robust, safe, and clinically relevant?
Join us for a practical webinar exploring research published in the Journal of Medical Imaging from the NeuroTest Project—a collaboration between deepc, Landshut University of Applied Sciences, Technical University of Berlin, and Germany’s National Metrology Institute. Our speakers will discuss their innovative MRI simulation framework, specifically designed to stress-test the reliability of AI segmentation networks under realistic variations in MRI imaging (T2-weighted FLAIR protocols).
Hear directly from Mehmet Yigitsoy (Head of AI Labs at deepc), Prof. Dr. Stefanie Remmele (Head of Medical Technology Research Group at Landshut University), and Yigit Avci (PhD student at King's College London) as they share how simulation-based validation can proactively identify weaknesses in AI models ultimately leading to safer, more effective patient care.
Join the conversation and discover how rigorous simulation testing can transform AI trustworthiness in clinical radiology.
Agenda
Identifying AI Weaknesses: Why MRI Acquisition Shifts Impact Clinical Reliability
MRI quality and imaging protocols vary across clinical institutions, directly impacting AI segmentation results. Learn how the NeuroTest Project developed a simulation-based framework to evaluate how reliably AI performs across realistic MRI variations. Understand the clinical importance of identifying unsafe imaging parameters before they impact patient outcomes.
Validating the Framework: Ensuring Clinical Realism in AI Testing
An accurate simulation is vital for meaningful testing of AI robustness. This session explores the comprehensive validation methods employed including clinician assessments, uncertainty analyses, comparative texture studies, and volunteer imaging to ensure simulated MRI images closely match clinical realities. Learn why thorough validation is essential to building confidence in AI performance.
Practical Collaboration: Safely Integrating AI into Clinical Practice
Effective AI adoption demands close collaboration between technologists, researchers, and radiologists. This session covers essential practical advice for healthcare professionals considering AI deployment, including the importance of transparency, continuous monitoring, patient safety considerations, and avoiding application bias. Explore best practices for involving radiologists early and consistently in AI development to ensure solutions genuinely improve clinical workflows and patient outcomes.
Speakers
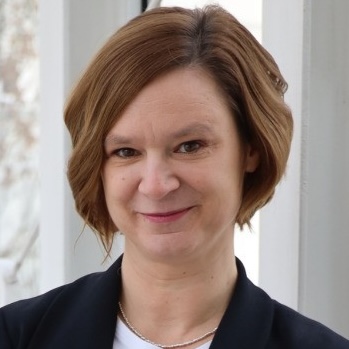
Stefanie is an expert in medical imaging and image processing for diagnostic and therapeutic applications. She studied Electrical Engineering at the KIT Karlsruhe, Germany, specializing in image processing, and completed her PhD at Philips Research Laboratories, Hamburg in collaboration with the KIT under the supervision of Prof. Dr. Olaf Dössel. Her research during her PhD centered on radial MRI, laying the foundation for her expertise in medical imaging. After her PhD, she continued her career at Philips Research, where she worked as a scientist and later as a senior scientist, driving innovations in imaging technologies. Now a professor of medical technologies at the University of Applied Sciences, she leads the research cluster Medical Technologies in Landshut. She has been involved in numerous research projects and is focusing on the synergy of rule-based and AI-driven methods for medical imaging in diagnostics and therapy. With her expertise and hands-on experience, Stefanie is passionate about bridging the gap between cutting-edge AI techniques and practical applications in healthcare, pushing the boundaries of technology to improve medical diagnostics and treatments.

Mehmet Yiğitsoy is an expert in image processing, computer vision, and artificial intelligence, having earned his PhD degree from the Technical University of Munich (TUM). He currently leads the AI labs team at deepc GmbH, overseeing academic collaboration projects and focusing on AI orchestration, evaluation, business intelligence, and NLP. Mehmet has also contributed to advancements in healthcare technology at various healthcare companies including Konica Minolta, Carl Zeiss Meditec, and Definiens, specializing in developing AI and image processing algorithms. His work is characterized by interdisciplinary collaboration and a commitment to advancing healthcare technology, underscored by numerous publications in leading scientific journals.
Mehmet Yigit Avci is a PhD student at King’s College London supervised by Dr. Jorge Cardoso, and funded by DRIVE-Health and deepc, specializing in AI-driven solutions for healthcare applications. His research focuses on developing smart orchestration systems. He leverages foundation models and representation learning to harness multi-modal radiology data for building scalable and robust frameworks. Yigit holds a master’s degree in biomedical computing from the Technical University of Munich and a bachelor’s degree in electrical and Electronics Engineering from Boğaziçi University, Istanbul. His prior research spans unsupervised learning, diffusion MRI, and enhancing the robustness of AI algorithms in medical imaging, with a particular focus on Alzheimer’s disease analysis and resilience in clinical workflows. Passionate about solving real-world healthcare challenges, Yigit is dedicated to advancing biomedical engineering through cutting-edge research that bridges AI innovation and patient care.